.png?width=2000&height=1125&name=GT%20Webinars%20Spring%20-Fall24%20(14).png)
-
Fault detection and predictive maintenance of complex physical systems are now achievable in the Industry 4.0 era due to the wealth of monitored data
-
Multi-physics simulation models of reciprocating compressors in Digital Twin Environments allow for the quick generation of synthetic training datasets and specific labelled anomalous datasets.
-
Thanks to machine learning it is now possible to reproduce and prevent faults without risking damage to expensive machines.
Abstract
In this webinar, we will talk about the advancements in fault detection and predictive maintenance for complex physical systems in the era of Industry 4.0. With the vast amount of data available today, there is a growing potential to effectively predict and prevent issues in industrial machinery. Multi-physics simulation models of reciprocating compressors as part of Digital Twin Environments have advanced significantly, thus allowing for the quick and easy generation of synthetic training datasets and specific labelled anomalous datasets simulating real-world faults. We will talk about a well-known commercial multi-physics simulation software that is used to model an industrial reciprocating compressor system with various domains including bearings, valves, compressor shaft mechanics, NVH, lubrication, and gas flow dynamics. The model is exercised with variability of typical physical parameters to simulate real-world operation of well-running, non-faulty reciprocating compressor systems and generate “no-fault” virtual input datasets. Faults are then intentionally introduced within the digital twin system to simulate real-world anomalies manifested via behavioral changes in signals of typical sensor data (e.g., compressed gas pressure) present in actual compressor systems. Supervised learning techniques are applied to train and optimize machine learning models for fault detection. The trained models can be deployed in real-world scenarios with online real-time monitoring to detect potential failures and identify fault causes. This can help with predictive maintenance of compressor systems, reduce unscheduled downtime, and thereby decrease the economic impact from loss of production. The promise of such technology is demonstrated as the ability to reproduce and prevent faults without actually creating them on a real machine, which would risk severely damaging these large and expensive machines.
Overall, this webinar will demonstrate the promise of technology in reproducing and preventing faults without risking damage to expensive machinery.
Topics include:
• Thanks to the abundance of monitored data, fault detection, and predictive maintenance of complex physical systems is now possible in the Industry 4.0 era.
• Multi-physics simulation models of reciprocating compressors in Digital Twin Environments allow for the quick generation of synthetic training datasets and specific labelled anomalous datasets.
• Supervised learning techniques are applied to train machine learning models for fault detection.
• Trained models can be deployed in real-world scenarios for online real-time monitoring to detect potential failures and identify fault causes.
• This technology can help with predictive maintenance of compressor systems, reduce unscheduled downtime, and decrease the economic impact from loss of production.
• A significant advantage of this technology is the ability to reproduce and prevent faults without risking damage to expensive machines.
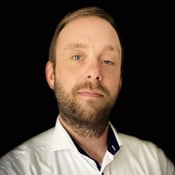
Nils Framke | Presenter
Senior Applications Engineering Manager, Thermal Fluid Systems | Hydraulics Lead at Gamma Technologies GmbH